
In the aid industry, the facts don’t match the wishes
The correlation between development aid and economic growth is close to zero. This poses not only challenges in terms of policy, but also in terms of ethical standards in science.
Lesen Sie die deutsche Version hier.
It is a tough old question: Does development aid generate development? Translated into the language of economics it becomes: Has aid to the poor countries generated economic growth? This is known as the aid effectiveness question. It is analyzed in a large literature.1 The reason why new papers keep coming is simple: We all want aid to work and alleviate world poverty, but the findings in the literature are weak and unstable.
Technically, aid effectiveness is an easy question to analyze. The statistical techniques for growth regressions are well known, and the data are ideal. Since aid programs started 60 years ago, almost 10’000 pairs of annual observations for aid and growth have been published. The average aid share is about 7 percent of gross domestic product (GDP) in the recipient country.
From similar data in other fields, 10 other factors have been shown to have a robust impact on growth. When comparable, most of these factors are of a smaller magnitude than 7 percent of GDP. If aid gave development, it should be easy to show – but it is not. By now, this is well known in development research.2
The basic observation in the field is the zero correlation that results if one looks at two data sets: One is the aid-shares (of GDP) of the recipient countries and the second the real growth rate of the same countries. The two data sets might be averages for 5 of 10 years and they may be shifted relatively to give aid some time to work. However, the correlation is always close to zero, and sometimes even negative.
If the discussion of aid effectiveness were a normal scientific one, it would be boring as it would deal with the second decimal only: Is there a tiny effect or none at all?
On the gravy train
However, the discussion of aid effectiveness is far from boring. Hot scientific disagreement is often brought about by something else that gives it zest. In the case at hand, the controversy is due to two strong priors: The first ist the angel prior: Aid aims at doing good, and we all want to be on the side of the angels. The second is the gravy prior: Aid has now reached $160 billion. About 10 percent is fees to consultants, including most development economists.
The two priors point in the same direction – the angel prior gives the researcher a moral justification for gravy seeking. Hence, research in aid effectiveness is a prior-ridden field where the tension between the priors and the basic fact has generated a large literature.
The aid effectiveness literature is embarrassing seen in the perspective of research ethics. It ought to be the job of research to explain the facts – not to explain away the facts. When our priors desire a result, we should be extra careful to make sure it holds. However, this is not always the case in this field. The following is a short summary of the results of 300 studies in the aid effectiveness literature. Anyone who tries to look beyond the priors ought to agree on these.
The micro-macro paradox
On the micro level, it is uncontroversial that about 50 percent of all aid projects succeed3; but development is a macro level phenomenon. The macro aspect is built into aid programs through social cost-benefit analyses. For many years, the criterion for determining if an aid project was acceptable was that it would contribute at least 10 percent to growth. The 50 percent success rate means that half of the projects contribute more than 10 percent, and the other half less; so we expect that 10 percent hold on average. If it does, projects amounting to 7 percent of GDP should generate 3/4 percentage points of growth per year. This is almost half the growth of the average less developed country.
Some aid is given for reasons unconnected to economic development, e.g. as emergency aid, aid to democracy and culture, aid to the «good» side in conflicts, aid to help countries making peace after conflicts, to help refugees, etc. Even if we say that only 2/3 of aid is aimed at development, it should still contribute about 1/3 of the growth in the average poor country. This should be easy to show – but it’s not visible. The micro findings for the individual projects and the macro result for the whole society are thus in contrast. This is known as the micro-macro paradox of development aid.4
The tension between the zero-correlation result and the two priors has generated the large literature in the field. Here the reader may think of the old saying: If you torture the data long enough, it will confess!
The reluctancy bias
The torture of the data is done by large-scale data mining. Scores of a thousand models have been applied to the data by the full battery of statistical techniques. Many models have been estimated for each one published.
Growth regression is a field where it is notoriously easy to make model variants. Every researcher has a computer with a fine statistical program ready to run. With these powerful tools, he can easily estimate 1000 model variants. Each estimate provides one value of aid effectiveness. We know that approximately 950 of these estimates are insignificant. However, the remaining 50 are significant, and of these about 25 are positive. What happens if we choose the one that is the most positive? It will make everybody happy, but maybe we have all been conned?
A careful data search is a fine way to propose new regularities. However, new models discovered by data mining must be independently replicated (that is, by new authors on new data) to be trustworthy. This is well known by researchers in medicine, physics, etc., but in the social sciences, it is only accepted in principle – not in practice.
While we are searching the 1000 model variants, the priors enter. Researchers must have publications to prosper. The 950 insignificant results are difficult to publish, and 25 results have the «wrong sign» – all insiders will reject them. Therefore, it is tempting to steer the search process away from them too.
Hence, the attention of the researcher will somehow concentrate on the 25 good models, and on finding reasons why the best of these nice models are true. The very word research refers to the process whereby you search and search again. A research result happens when a researcher stops searching. When does he stop searching? Surely, he stops when he is satisfied with the result. It is hard to believe that the two priors do not influence when satisfaction sets in. Hereby I do not suggest that researchers are dishonest, only that they are human.
The two priors thus cause the search process to be skewed – leading to biased results – and in the field of aid effectiveness both priors are in the same direction. This has caused Chris Doucouliagos and me to suggest that this literature may suffer from a reluctancy bias. The profession is reluctant to publish negative and insignificant results.
A set of statistical tests has been developed to check if a literature has a bias like that. When these tests are applied on the aid effectiveness literature, all the red warning lights come on – and confirm our suggestion.
The question of causal direction
The gut reaction of any economist, looking at the zero-correlation observation, is that it does not sort out causality. We want to study if aid causes growth, but what we observe may reflect the reverse causality that low growth attracts aid. Maybe the world is so mischievous that the two causal links have the reverse sign and the same size. It would surely be a strange coincidence, but it has been thoroughly researched.
The causal factors behind an observed empirical regularity is always a key question, and a large, and highly technical, field of causality tests have been developed. The techniques developed have been applied to the aid-growth literature.
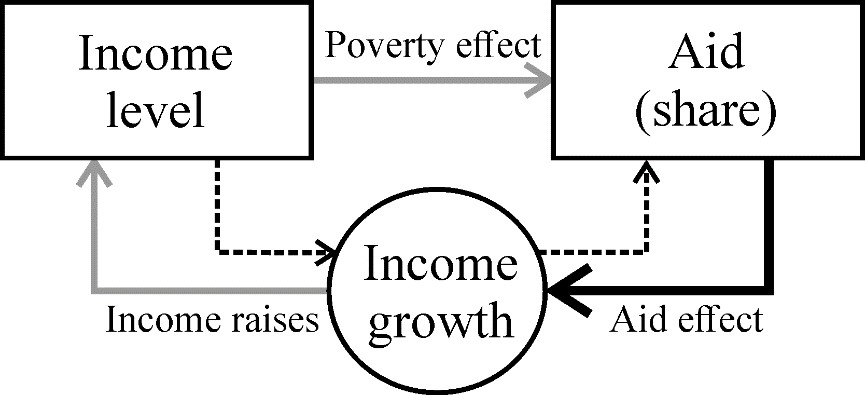
Figure 1 shows the causal links that may potentially be involved. It includes two effects: The aid effect is the effect of aid on growth discussed. The poverty effect is that high income levels reduce aid flows. If aid works, it raises growth; the income level increases, causing aid to be reduced. Thus, if the two gray arrows on the figure were strong there would be reverse causality in the aid effectiveness relation, so that the estimated effectiveness effect becomes too small. However, this possibility is small, as the effects are small within a time horizon of 10 years. An extra growth of e.g. 1 percent per year in 10 years increases the income level by 10.5 percent only. This will only reduce the aid level marginally.
There are two additional effects on aid (the dashed lines in the figure), which are both found to be small. First, growth is higher in middle-income countries, but only marginally so.5 Second, higher growth can increase aid because countries with faster growth have more projects with high benefits/costs ratios, so it is easier to find good projects to support with aid. In addition, commercial interests may argue for aid flows to countries that are on their way to become future markets. These effects run counter to the poverty effect. When all these weak effects are added, it is likely that most of the effects cancel out to leave a really small net effect. This is precisely what the literature finds.
Researchers are human
The aid effectiveness literature has reached 300 studies. At least 200 man-years of research have been put into this production. Therefore, it is worth taking seriously.
Meta-analysis studies the distribution of the published results in a literature about the same effect, such as the effectiveness of aid. It studies if the results converge to the same results, if there are breakthroughs in the research, and in addition, it has developed tests to detect publication biases. A publication bias (such as the reluctancy bias) means that the average of the published results deviate systematically from the average of the estimated results.
Six meta-studies have been made in the field of aid effectiveness.6 We have found 1777 estimates of the effect of aid on growth, which are so well documented that they can be converted to the same scale. When their distribution is analyzed, we find that it has two properties that are unreasonable if we assume that researchers are robots programmed to seek truth only.
Results ought to become clearer (more stable and significant), the larger the data set on which they are estimated; but (1) results get smaller and less significant, the larger the data set. The aid industry should learn from experience, as everybody else, so the effectiveness should increase, but (2) the estimated effect of aid falls over time. Overall, we do find a small positive effect, of which half is publication bias; thus, the effect is negligible.
The two unreasonable results turn reasonable if we take the reluctancy bias into consideration. The variation of results falls, the larger the data set is, and the data set grows over time. Thus, both the most positive and the most negative results fall as the number N of observations in the data set increases, but as the negative results are rejected anyhow, all we see is that the published estimates fall when N rises.
If you accept that researchers are human, everything hangs together. It is not the effect, but the bias that goes down, when more data are used. This makes it look as if aid effectiveness falls over time, but this is an illusion. When the distribution of the published results is adjusted for the reluctancy bias with the methods developed for this purpose, the average result becomes dubious. The literature has not found a robust method to reject the zero correlation result.
Another way to understand the implication of the reluctancy hypothesis is to consider the characteristic fate of the most aid-positive models. When a new such model is found, it is easy to publish, and it is heavily cited and popularized. It is received with great joy by the aid industry: Finally, it has been shown that aid works! The diligent researcher who found the model is promoted, etc. But then after three years, new data appear, and the model collapses, and it is quietly swept under the carpet to all the others.
Thus, we have to conclude that the reason for the zero correlation result is that both links are basically zero. This raises two questions. The first question is if there are effects that escape the methods used. Think of aid that increase the quality and quantity of teachers. It affect the economy when the pupils educated by the better teachers leave the schools and proves to work better! That may take 15 years, but then the effect will be present for the next three to four decades. Such effects are notoriously difficult to catch by growth regressions. A dam may take 10 years to build, but then the power generated may be absorbed in the next few years. The effect of this may be easier to catch, but it still needs long lags. Thus, it is possible that some of the effect of aid escape our attempts to catch the effects.
The second question is why does aid have such a small effect? I have found three answers to that question: One is termed fungibility: Aid often finances projects that would be made anyhow, and thus countries can afford other projects. These other projects, however are unlikely to be equally good. The second explanation is termed Dutch Disease. Aid causes an inflow of foreign currency so that it becomes cheaper, and thus foreign goods become cheaper and domestic goods more expensive. This harms the development of an export industry. The third effect is that aid projects require a great deal of executive capacity in the recipient country, and if this is scarce, other projects suffers.
The aid industry
As mentioned, the development aid industry has now reached an annual turnover of $160 billion. It is a complex mixture of public agencies and private firms which has many links to research and media. Very few in development research are without connections to this industry. Thus, we have all interests in the continuation of the industry. And there is also the angel prior.
It is a fascinating experience to discuss development aid, for here one inevitably encounters these interests. Many discussants live fully or partly of aid. One of the most common arguments is that one should go easy on the facts of aid effectiveness as it may harm aid if the facts become more known. This point of view is also common from people in research positions. They thus reveal that they feel obliged to tailor their research to be as positive to aid as possible. This is why the aid effectiveness literature has the reluctancy bias.
Today, research is increasingly financed by sponsors. Like everybody else working at a university, I like sponsors. Sponsors have interests – this also applies to public sponsors. It is why ethical standards for research are becoming increasingly important. They demand that researchers with economic interests in a field state this clearly when they publish in the field.
I have studied 100 articles on aid effectiveness and tried to check who the researchers are. Many report only a university affiliation.7 However, if you look on their web site or use a search engine, a surprising number turn out to have an extra job in the aid industry. I have even found cases of authors who had special research positions financed by aid budgets but did not mention this curious coincidence in their papers on aid effectiveness.
Thus, the reason why the zero correlation result occurs is simple: It is because the two causal links between development aid and development are both close to zero. In spite of good data and large research efforts, it has not been proven that development aid has a substantial effect on development.
This does not mean that there is no effect of aid at all. There may be good social consequences, though it doesn’t appear that they affect national levels. Furthermore, we know that aid can alleviate emergency situations, food aid can feed the hungry, military aid can help the good guys defeat the bad ones, etc. Development is something else. It is not easy to generate, and it is notoriously difficult for foreigners to give a country a treatment to make it grow.
For all of this my article has a sad message. I think that anyone who has seen the poverty in the less developed countries will agree with me: It would have been much better if aid had been effective.
Martin Paldam is Professor emeritus of Economics at the University of Aarhus. He is specialized in development economics. His most recent book is «The Grand Pattern of Development and the Transition of Institutions» (Cambridge University Press, 2021).
See Hristos Doucouliagos and Martin Paldam: The aid effectiveness literature. The sad results of 40 years of research. Journal of Economic Surveys 24(1), 2009, p. 1-24; Hristos Doucouliagos and Martin Paldam: Finally a breakthrough? The recent rise in the size of the estimates of aid effectiveness. In: B. Mak Arvin and Byron Lew (eds.): Handbook on the Economics of Foreign Aid. Cheltenham: Edward Elgar, 2015, p. 325-349. ↩
Barro and Sala-i-Martin’s leading textbook on growth and development contains about 100 pages on the factors causing growth. It does not even mention aid (see Robert J. Barro and Xavier Sala-i-Martin: Economic growth. Cambridge: MIT Press, 2004). The same applies to the four large volumes of the Handbook of Growth (see Philippe Aghion and Steven N. Durlauf: Handbook of Economic Growth Amsterdam: North-Holland, 2014). Even in the four heavy volumes of the Handbook of Development (Jere Behrman and T.N. Srinivasan: Handbook of Development Economics. Amsterdam: North-Holland, 1995), aid plays a small role. ↩
Robert Cassen: Does Aid Work? Oxford: Oxford University Press, 1994. ↩
Paul Mosley: Aid-effectiveness: The Micro-Macro Paradox. In: IDS Bulletin 17, 1986, p. 22-27. ↩
Martin Paldam: The Grand Pattern of Development and the Transition of Institutions. Cambridge and New York: Cambridge University Press, 2021, Chapter 11. ↩
See Doucouliagos and Paldam 2008 and 2016. ↩
I should mention that I have worked for the United Nations Development Programme, and been consultant for the World Bank and the Interamerican Development Bank, though it is a dozen years ago. ↩